Are resumes really read by people?
Every once in a while, applicants ask us questions about our recruitment process. They seem to be particularly interested in the preliminary screening that is based on resumes. This is probably the most “secretive” step of the process, because the preliminary screening criteria tend to remain obscure to the applicants, unless the applicants ask about them actively. Many applicants wonder if we use automation for the preliminary screening and if resumes are really read by people. Yes, they are, every time – at least at MPS.
The debate around recruitment algorithms is heated, but in my opinion, humans are still better equipped for recruitment than machines. There are many arguments that support the use of automation, but there are two sides to everything, as I will discuss below.
Automatic application handling saves the consultant’s time.
This is definitely true. However, this can also be approached from a slightly different angle: we believe that a carefully prepared recruitment announcement brings time savings. Describing the job and the recruiting organization realistically and expressing the properties required of the applicant clearly are surprisingly effective ways to eliminate irrelevant applications. Resumes and applications that have nothing to do with the job description are extremely rare. The majority of resumes we read are either relevant or highly relevant for the job.
Humans make more errors than machines.
True. But humans also recognize excellent candidates better among good candidates, and we see this is as an essential ability in successful recruitment. Machines are less adept at seeing the difference between excellent and good – at least for now. In theory, a machine could learn this, but in order to create sufficiently accurate analyses, the algorithm would need a huge number (at least hundreds) of previous resumes and applications that are relevant, fairly relevant and not-so-relevant for the position in question. In practice, this is not feasible at the moment.
Machines cannot be tricked by applicants.
That is true, but they can be tricked by data. An algorithm needs a lot of data to make a valid decision and often, data is not available in sufficient amounts. This problem can be avoided by using data from secondary sources, such as behavioral data from social media. However, this creates a new problem: the decision is, to a large extent, based on data that is irrelevant for the position. I see this as one of the biggest problems in automated recruitment.
Assessments by humans are always subjective. Algorithms are more objective.
True again, but there are many cures to the problem of subjectivity. An experienced and trained consultant recognizes the sources of subjective interpretation and strives to assess candidates as objectively as possible. However, using multiple methods is most important: information must be obtained from multiple sources. This is why we use a range of tests to evaluate candidates, in addition to interviews. This reduces the importance of subjective interpretation significantly, and personally, I believe that despite their subjectivity, humans can create a much more accurate and multifaceted profile of the candidate than algorithms, when the evaluation is done well.
In the future, algorithms will be able to make more accurate suitability decisions than humans, because an algorithm bases its decision on big behavioral data instead of personal observations and interpretations.
Algorithms excel in making calculations with the help of big data. In my view, they could be really helpful, for example, for physicians who make diagnoses by applying various alternative diagnoses to the symptoms. This takes quite a lot of probability calculations, which a machine can perform within seconds. The evaluation of persons is also based on hypotheses, but when we evaluate a person’s behaviour or style of working, we are dealing with more abstract and complex matters. For example, humans are better than machines at evaluating interaction skills, and I believe this will not change for a long time.
I see the role of technology in recruitment as that of an assistant supporting the overall process. Of course, algorithms have many benefits – most importantly, they enable huge time savings. For example, machines can screen applicants to identify those who do not meet the job-specific profile at all, which saves time. In the case of positions that are frequently open for lots of applicants, the algorithm could be taught to recognize a candidate’s experience profile or test profile more accurately, if the candidate pool is large enough for screening on the basis of experience and test results. Machines could also learn the “cross interpretation” of test results, after which the algorithm’s interpretation could serve as an objective “second opinion” to support the interpretation of the consultant. I would never give responsibility for the entire recruitment to an algorithm under any circumstances, and I do not see this as realistic in the future.
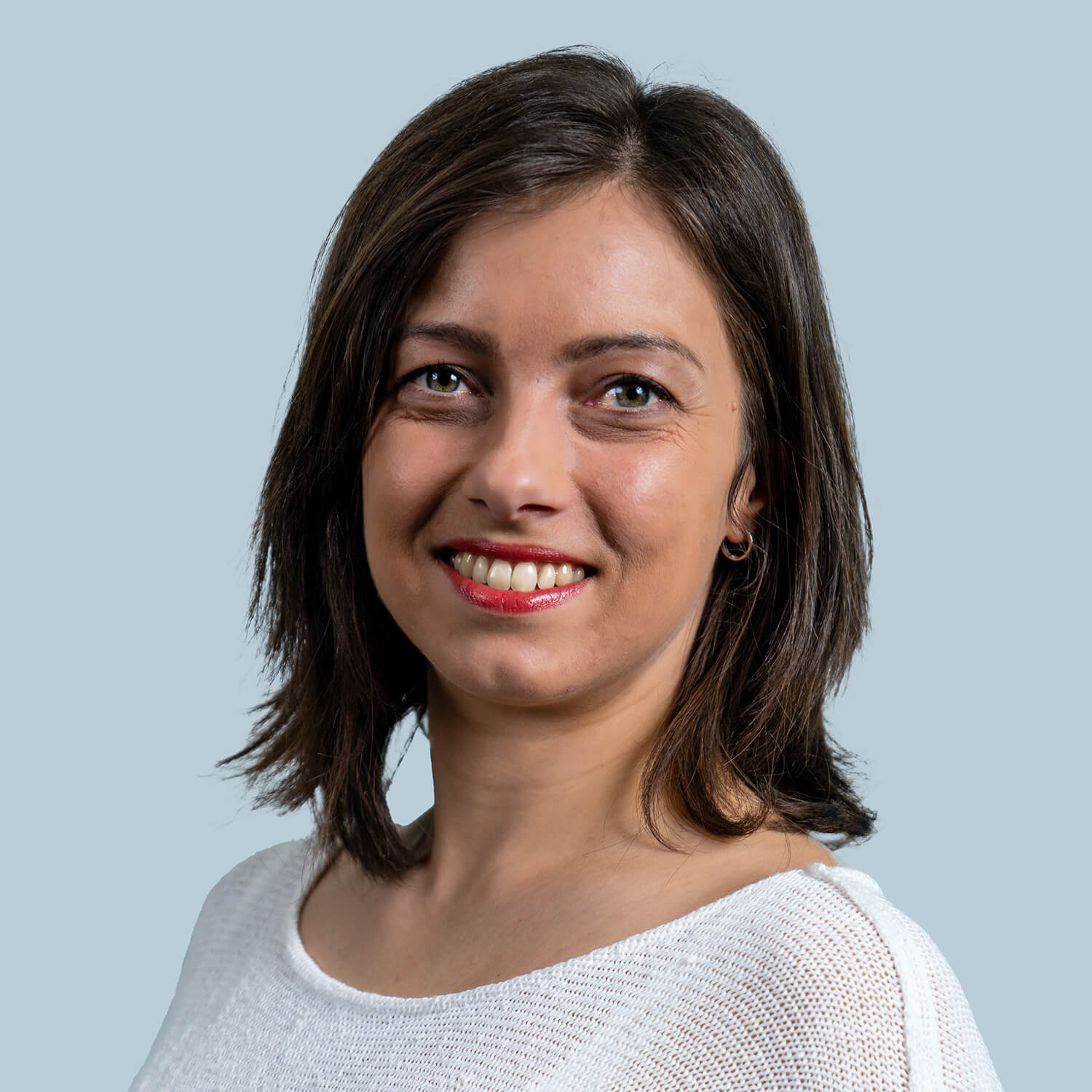